Prediction of Exacerbation Events using myCOPD data.
10 March, 2022
This is a subtitle for your new post
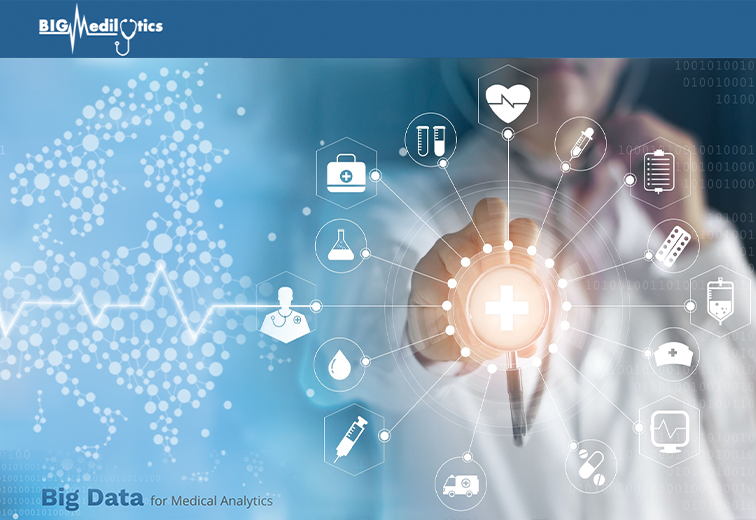
As Pilot 4, my mhealth and the University of Southampton collaborated to develop a machine learning model to predict and manage worsening of chronic obstructive pulmonary disease (COPD), termed exacerbation. Results demonstrated that self-reported COPD data, using a digital health app, can be used to identify users at risk of exacerbation within 3 days with moderate discriminative ability
Prediction of Chronic Obstructive Pulmonary Disease Exacerbation Events by Using Patient Self-reported Data in a Digital Health App: Statistical Evaluation and Machine Learning Approach.
BigMedilytics (Big Data for Medical Analytics) was the largest EU-funded initiative to transform the region’s healthcare sector. Its aim was to enhance patient outcomes and increase productivity in the health sector by applying big data technologies to complex datasets while ensuring security and privacy of personal data.
Twelve pilots across eight countries, more than 11 million healthcare records, and data from other sectors including insurance and public bodies addressed three themes: Population Health and Chronic Disease Management, Oncology and Industrialization of Healthcare Services; and covered the entire Healthcare Continuum from Prevention to Diagnosis, Treatment and Home Care.
As Pilot 4, my mhealth and the University of Southampton collaborated to develop a machine learning model to predict and manage worsening of chronic obstructive pulmonary disease (COPD), termed exacerbation.
Using the cloud-based digital health application (app) myCOPD, analysis explored several in-app reported variables including medication usage, COPD assessment test scores, and symptom scores. This led to the development of both heuristic and machine learning models.
Results demonstrated that self-reported COPD data, using a digital health app, can be used to identify users at risk of exacerbation within 3 days with moderate discriminative ability (AUROC 0.727, 95% CI 0.720-0.735). Further research utilizing additional linked data (particularly from medical devices such as smart inhalers, physiological monitoring sensors, and environmental sensors) are expected to increase the accuracy of these models.
Data self-reported to health care apps designed to remotely monitor patients with COPD can be used to predict acute exacerbation events with moderate performance. This could increase personalization of care by allowing pre-emptive action to be taken to mitigate the risk of future exacerbation events.
With the Health and Social Care Secretary setting a target for 4 million to benefit from personalised care by March 2024, leveraging the data collected by these apps in prognostic models could provide increased personalization of care by allowing pre-emptive action to be taken to mitigate the risk of future exacerbation events
Findings have been published in the JMIR Medical Informatics:
©Francis P Chmiel, Dan K Burns, John Brian Pickering, Alison Blythin, Thomas MA Wilkinson, Michael J Boniface. Originally published in JMIR Medical Informatics (https://medinform.jmir.org/2022/3/e26499), 21.03.2022.
This is an open-access article distributed under the terms of the Creative Commons Attribution License (https://creativecommons.org/licenses/by/4.0/), which permits unrestricted use, distribution, and reproduction in any medium, provided the original work, first published in JMIR Medical Informatics, is properly cited. The complete bibliographic information, a link to the original publication onhttps://medinform.jmir.org/, as well as this copyright and license information must be included.
About my mhealth
my mhealth’s digital therapeutics have been prescribed to over 90,000 patients with chronic conditions, resulting in reduced morbidity and hospital admissions. It serves patients across a range of long-term conditions, including COPD, asthma, diabetes and cardiovascular disease. Real world and clinical trial evidence demonstrates the efficacy of digital interventions on the my mhealth platform.
For more information on my mhealth, visit www.mymhealth.com.
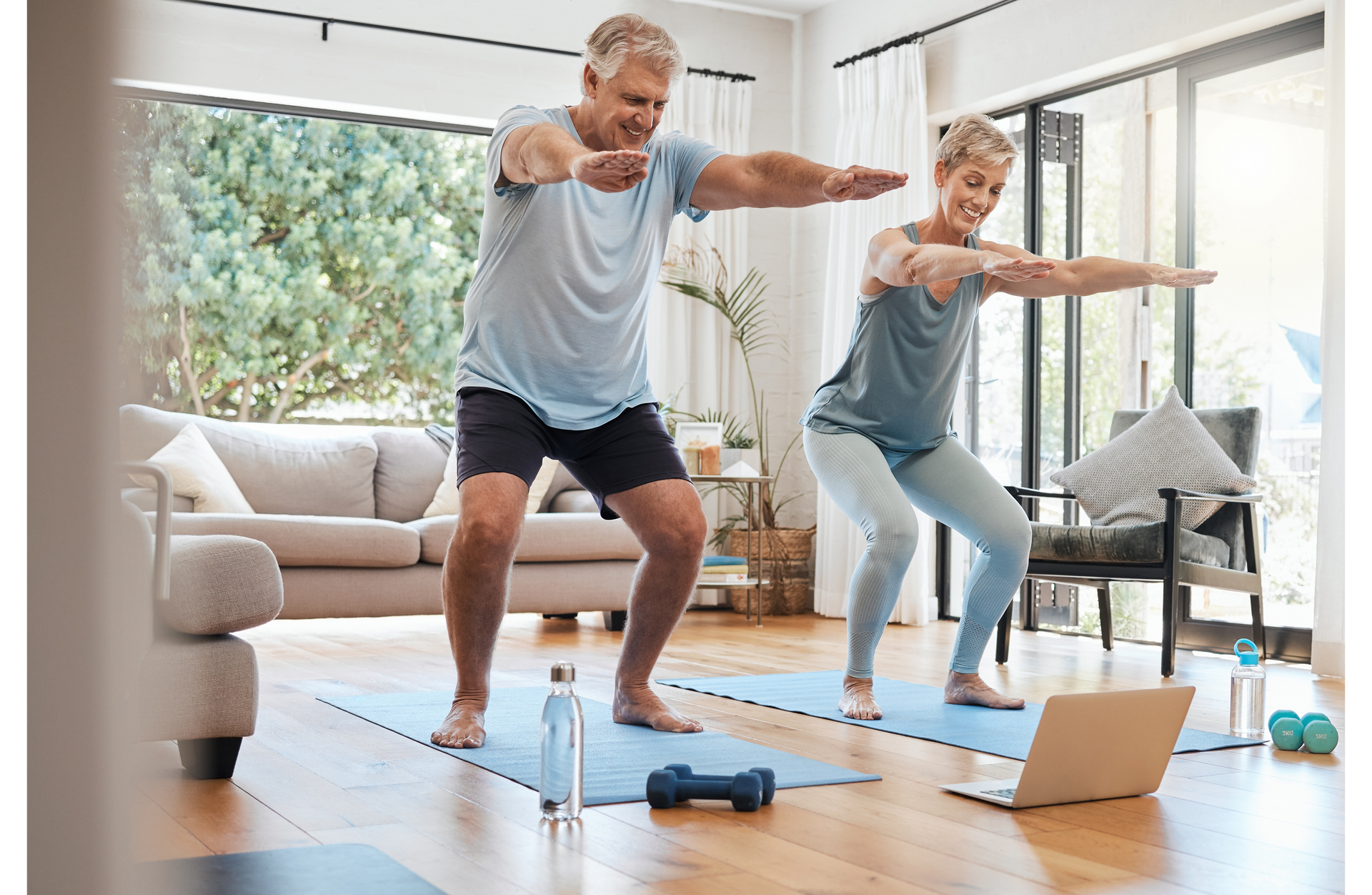
Cardiac rehabilitation is undergoing an evolution as health care systems embrace digitally enhanced programmes that promise to support patient and service outcomes. Cardiac rehabilitation (CR), a NICE recommended cornerstone in cardiovascular disease recovery and secondary prevention, has traditionally relied on in person sessions. However, there are often challenges to uptake and completion which can affect the number of people who successfully undertake and complete a programme. Digitally enhanced cardiac rehabilitation (DECR) is a modern approach that blends traditional care with digital delivery to move towards a menu-based approach to care. Leeds Community Health Care CR team were one of the early adopters of this approach, introducing the myHeart app into their pathways in 2020. ‘we started using the app as our face-to-face classes had stopped during COVID and this gave us the option for patient to access an exercise programme and cardiac information digitally. We have since restarted our face-to-face programmes, but we still offer the app as an option for our patients. It gives us wider complimentary range for our patients.’ N. Simpson - CR lead 5 years on and the team have excelled in their use of the app and have just reached the huge milestone of over 1000 patients now registered on myHeart. The way the team have utilised the app in their pathway has seen high levels of patient engagement with 84% of patients activating their account, competing over 9000 education sessions and over 12,800 activity diary entries. A recent patient survey showed the impact this is having on the patients: 92% rated the app as good or very good 85% found the apps easy to use 88% would recommend the app to others 77% said the apps have helped them to learn more about their condition 81% felt more confident in managing their condition since using the app 85% felt there has been an improvement in symptom control since using the app 81% intend to continue using the app. ‘the digital app gives our patients the choice of what they want their Cardiac Rehab to consist of and helps us make individualised programmes for our patients’ N. Simpson - CR lead Health services across the UK are working to leverage digital tools to support and enhance service delivery. Digitally enhanced cardiac rehabilitation offers services the chance to redefine recovery – offering more flexible, accessible care. For more information on this article or other my mhealth projects, please get in touch https://mymhealth.com/contact-us
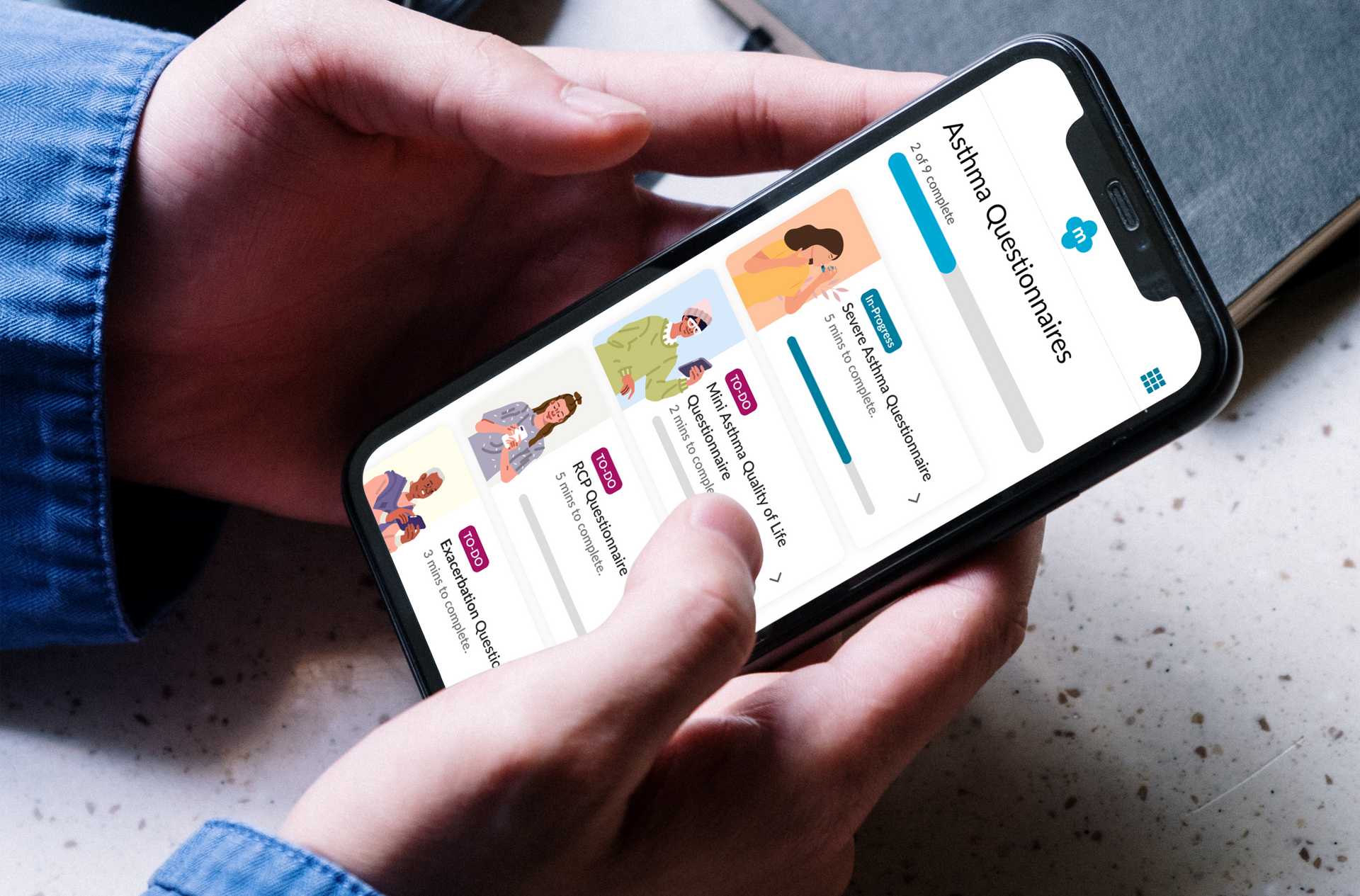
Empowering patients living with Severe Asthma: How severe asthma services are digitally transforming patient care. Living with severe asthma can be overwhelming. Frequent flare-ups, emergency health care visits and the burden of managing complex medication can take a toll – not just physically, but emotionally and mentally. Severe asthma services across the UK are working to address these challenges through the introduction of the myAsthma+ app, designed specifically to support patients with severe asthma in managing their condition more effectively and confidently. myAsthma+ combines high quality digital tools with clinical expertise to offer a range of tailored resources to support patient empowerment and improve self-management. Studies have shown that effective self-management can lead to reduced flare ups, improved quality of life and reduced unplanned health care demand (1). myAsthma+ puts evidence-based strategies into action, offering structured, easy to use tools that help patients take an active role in their care. Key features of myAsthma+ are: • Tailored education to improve health literacy • Self-management tools to track symptoms and support timely response to changes • A comprehensive series of mindfulness resources to aid stress reduction and anxiety management • Validated assessment tools to help patients and their clinicians in the management of their condition • And much more…. Over 3,300 patients have now been registered onto myAsthma+ across the UK. A recent patient survey* found that: • 84% found the app easy to use • 72% felt they had a greater understanding about their condition since using the app • 69% felt more confident in managing their condition
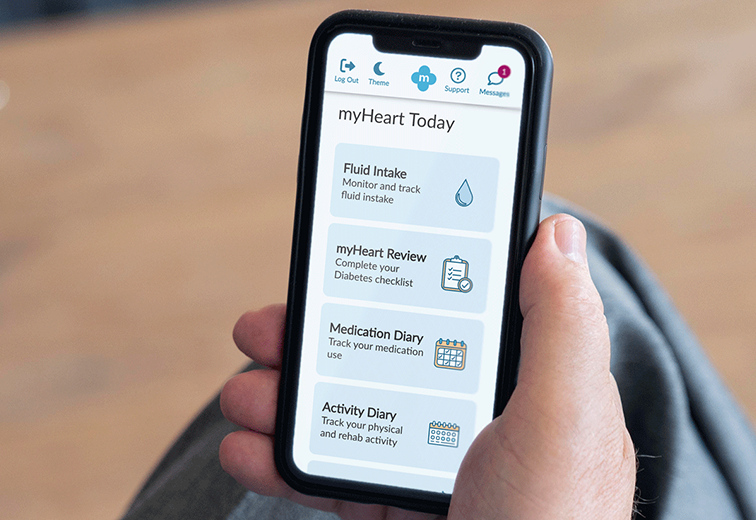
Results of a service evaluation using myHeart in a large London-based acute NHS Trust. We are pleased to report the outcomes from this recent service evaluation of myHeart and the potential benefit of using the myHeart app to supplement existing cardiac rehabilitation (CR). Cardiac rehabilitation is an essential evidence-based intervention that supports patient recovery following a cardiac event. It offers patients a structured education and exercise programme to aid recovery and support behavioural changes to help reduce the risk of future cardiac health complications. The myHeart app provides a structure educational and exercise intervention that mirrors current CR service delivery as well as supportive self-management tools. Overall, 721 patients were invited to participate in 1 of 4 groups (class-based CR, class-based CR with myHeart, home-based CR and home-based CR with myHeart). A total of 584 patients opted to use class-based CR and of these 43 chose to include myHeart to support them with this. There were 137 patients in the home-based group, with 54 choosing to include myHeart alongside their CR. This 12-week evaluation involved functional, physical and psychological assessments both before and after CR to explore potential changes. Patients were also asked to complete a rating of perceived exertion Borg RPE scale (Borg 6-20). Those in the home-based groups were contacted mid-way through the study. Results identified three key outcomes: 1. Blood pressure, cholesterol, LDL, BMI, HbA1c and exercise were all very similar across the groups with marginal differences across each measure. 2. Drop-out rates (DOR) of patients being invited to attend CR and attending CR were significantly lower in those groups with access to myHeart. * Class only: DOR = 58.2% Class + App; DOR = 25.6% * Home only: DOR = 73.5% Home + App; DOR = 42.6% 3. Those patients with access to myHeart and in the home-based group saw the greatest improvement in anxiety and depression scores. This real-world evaluation provides an encouraging insight into the potential impact of myHeart to supplement CR services, and is suggestive that, as an adjunct to support both class and home-based programmes, myHeart helps to reduce drop-out rates in CR and can assist in reinforcing continuous engagement with CR programmes.
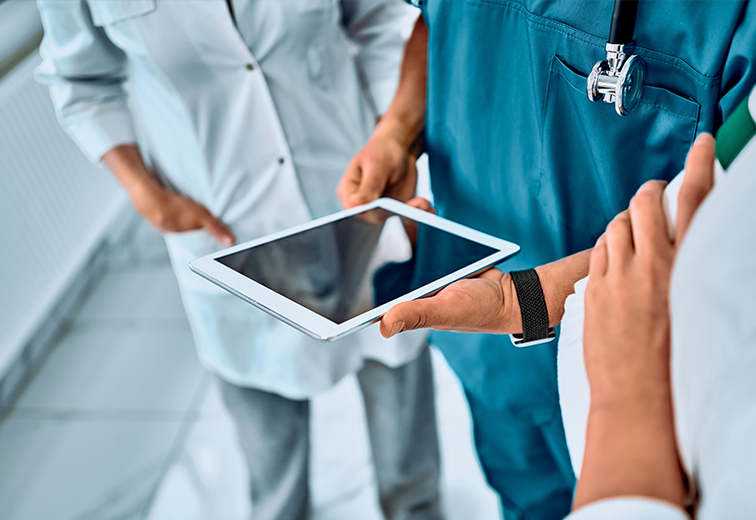
Results have led to continued QISMET Accreditation for myDiabetes (QIS2015) and have revealed the app could play an important role in supporting structured patient education delivery for type 2 diabetes following initial diagnosis, and as an ongoing resource. Together with a large Health and Care Partnership, we led a multi-centre service evaluation to explore the impact of myDiabetes on education course attendance rates. T2DM is a serious and growing problem worldwide and affects more than 3 million people in the UK. Structured education is a large part of managing T2DM to promote a healthy lifestyle and improve blood sugar control. However, the uptake for education courses has been less than encouraging across the UK. By offering a digital alternative or adjunct to a class-based course those who are unable or prefer not to attend a class-based programme, are able to receive structured education. myDiabetes is an app to support patients and clinicians manage diabetes together, remotely and at scale. Overall, 83 T2DM patients were recruited by the healthcare team, of which 28 chose to use myDiabetes alone, 35 chose only usual care, and 20 chose to use both. Patient education usage was monitored over a 12-week period. During this evaluation we monitored changes in diabetes related clinical health outcomes where possible, including HbA1c, blood pressure and body mass index. Participants in all three sites were asked to complete the Problem Areas In Diabetes (PAID) questionnaire at the beginning and end of the evaluation to explore markers of improvement in diabetes related distress. Results showed the app was acceptable in this care setting with 31 of 42 patients using it alone or as an adjunct to usual care. A total of 586 education videos were watched, on average each patient watched 22.5 (SD 19.6) videos. There was a reduction in PAID scores across all arms, with the app only arm showing the greatest improvement. Patients using myDiabetes showed the greatest improvement in HbA1c (-7.5 vs –4.4 mmol/mol), Systolic Blood Pressure (-12.2 vs +3.3 mmHg) and PAID score (-6.8 vs –5.2). In this real world evaluation myDiabetes performed better than published education course completion rates and resulted in significant improvements in HbA1c and PAID score compared to classed-based programs. This supports the use of myDiabetes to support the delivery of structured based education for patients with type 2 diabetes.
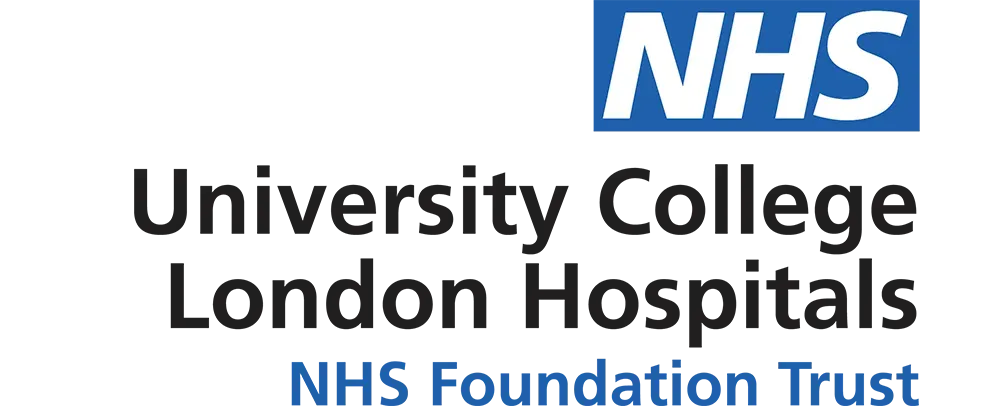
NHS University College London Hospitals NHS Foundation Trust, part of North Central London ICB, is taking a significant step towards enhancing patient empowerment and optimising disease management. Asthma is a chronic condition that affects millions of people worldwide, often leading to severe health complications if not managed properly. Recognising the critical need for effective self-management tools, NHS University College London Hospitals NHS Foundation Trust has chosen the myAsthma app to provide patients with the resources they need to take control of their health. Dr Kay Roy PhD FRCP, Consultant Respiratory Physician University College London Hospitals NHS Foundation Trust, comments “We are thrilled to introduce myAsthma as a self-management tool to our community. It represents a significant step forward in empowering our patients with asthma to take control of their health. By providing them with personalised support, we believe this tool will greatly improve their quality of life. Additionally, the use of myAsthma in outpatient settings will help triage patients more effectively, ensuring they are seen in a timely manner and appropriately referred for the right investigations and services. Our team is excited to see the positive impact this will have on the asthma population across North Central London ICB." The myAsthma app, part of the my mhealth suite of digital health solutions, is designed to empower patients with comprehensive tools and information to manage their asthma more effectively. Key features include: • Personalised Action Plans: Tailored asthma management plans based on individual patient needs. • Inhaler technique training: Contributing to better health outcomes and reduced risk of exacerbations • Medication Tracking: Reminders and logs to ensure patients take their medication as prescribed. • Symptom tracking: Easy-to-use tools for tracking symptoms and triggers. • Educational Resources: Access to a wealth of information on asthma, helping patients understand their condition and how to manage it. As more NHS partners embrace the my mhealth platform, we're thrilled to witness its growing impact and the positive changes it is bringing to long-term condition care. For more information on this article or other my mhealth projects, please get in touch https://mymhealth.com/contact-us
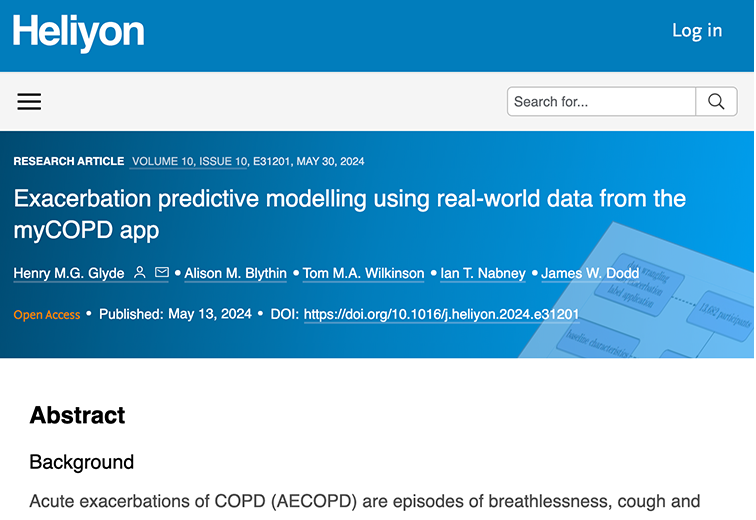
Henry M.G. Glyde1Alison M. Blythin2 Tom M.A. Wilkinson3Ian T. Nabney4 James W. Dodd5 EPSRC Centre for Doctoral Training in Digital Health and Care, University of Bristol, Bristol, UK my mHealth Limited, Bournemouth , UK my mHealth and Clinical and Experimental Science, University of Southampton, Southampton, UK School of Engineering Mathematics and Technology, University of Bristol, Bristol, UK Academic Respiratory Unit, Translational Health Sciences, Bristol Medical School, University of Bristol, Bristol, UK Abstract Background Acute exacerbations of COPD (AECOPD) are episodes of breathlessness, cough and sputum which are associated with the risk of hospitalisation, progressive lung function decline and death. They are often missed or diagnosed late . Accurate timely intervention can improve these poor outcomes. Digital tools can be used to capture symptoms and other clinical data in COPD. This study aims to apply machine learning to the largest available real-world digital dataset to identify AECOPD Prediction tool which could be used to support early intervention improve clinical outcomes. Objective To create and validate a machine learning predictive model that forecasts exacerbations of COPD 1-8 days in advance. The model is based on routine patient-entered data from myCOPD self-management app. Method Adaptations of the AdaBoost algorithm were employed as machine learning approaches. The dataset included 506 patients users between 2017-2021. 55,066 app records were available for stable COPD event labels and 1,263 records of AECOPD event labels. The data used for training the model included COPD assessment test (CAT) scores, symptom scores, smoking history, and previous exacerbation frequency. All exacerbation records used in the model were confined to the 1-8 days preceding a self-reported exacerbation event. Results TheEasyEnsemble Classifier resulted in a Sensitivity of 67.0% and a Specificity of 65% with a positive predictive value (PPV) of 5.0% and a negative predictive value (NPV) of 98.9%. An AdaBoost model with a cost-sensitive decision tree resulted in a a Sensitivity of 35.0% and a Specificity of 89.0% with a PPV of 7.08% and NPV of 98.3%. Conclusion This preliminary analysis demonstrates that machine learning approaches to real-world data from a widely deployed digital therapeutic has the potential to predict AECOPD and can be used to confidently exclude the risk of exacerbations of COPD within the next 8 days. Permission to use received from Henry Glyde. Read more on Heliyon website.
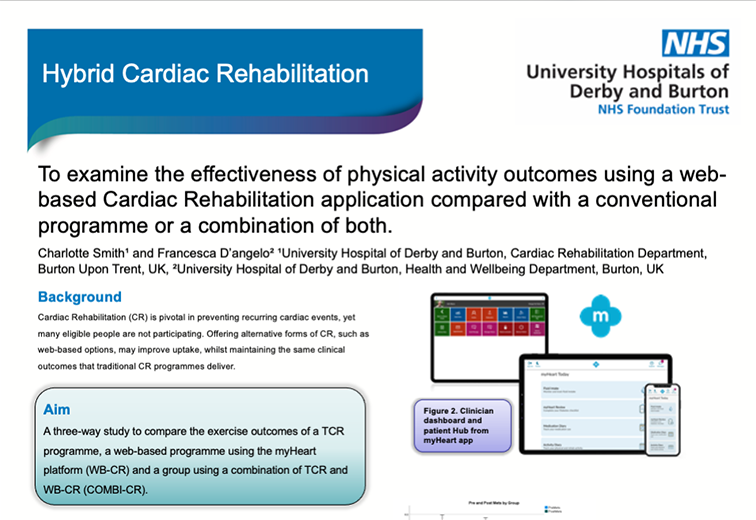
Charlotte Smith 1 Francesca D’angelo 2 University Hospital of Derby and Burton, Cardiac Rehabilitation Department, Burton Upon Trent, UK. University Hospital of Derby and Burton, Health and Wellbeing Department, Burton, UK To examine the effectiveness of physical activity outcomes using a web-based Cardiac Rehabilitation application compared with a conventional programme or a combination of both. University Hospitals of Derby and Burton NHS Foundation Trust poster presented at the BACPR Annual Conference October 5-6th 2023 Permission to use received from Charlotte Smith